Purrr Cheat Sheet
This code have been lightly revised to make sure it works as of 2018-12-18.
Cheat Sheet: purrr (5:16) Setup: Iteration with purrr (File Download) (3:39) purrr primerrr, Part 1: Reading Many Excel Files in a Directory (3:08). View purrr cheatsheet.pdf from STAT 1600 at Western Michigan University. Apply functions with purrr:: CHEAT SHEET Apply Functions Map functions apply a function iteratively to each element of a.
Purrr tips and tricks
If you like me started by only using map()
and its cousins (map_df
, map_dbl
, etc) you are missing out a lot of what purrr
have to offer! With the advent of #purrrresolution on twitter I’ll throw my 2 cents in in form of my bag of tips and tricks (which I’ll update in the future).
First we load the packages:
loading files
Multiple files can be read and combined at once using map_df
and read_cvs
.
Combine with list.files
to create magic1.
combine if you forget *_df the first time around.
If you like me sometimes forget to end my map()
with my desired out put. A last resort is to manually combine it in a second line if you don’t want to replace map()
with map_df()
(which is properly the better advice, but can be handy in a pinch).
name shortcut in map
provide “TEXT” to extract the element named “TEXT”. Follow 3 lines are equivalent.
works the same with indexes.2
use {} inside map
If you don’t know how to write the proper anonymous function or you want some counter in your map()
, you can use {}
to construct your anonymous function.
Here is a simple toy example that shows that you can write multiple lines inside map
.
This can be very handy if you want to be a responsible (websraping) pirate3.
discard, keep and compact
discard()
and keep()
will provide very valuable since they help you filter your list/vector based on certain predictors.
They can be useful in cases of webcraping where certain lines are to be ignored.
Where we here scrape Top Box Office (US) from IMDb.com and we use keep()
to keeps all lines that end in a integer and discards()
to discards all lines where the integer is more then 5.
compact()
is a handy wrapper that removed all elements that are NULL.
safely + compact
If you have a function that sometimes throws an error, warning or for whatever reason isn’t entirely stable, you can use the wonder of safely()
and compact()
. safely()
is a function that takes a function f()
and returns a function safe_f()
that returns a list with the elements result
and error
where result
is the output of f()
if it is able to run, and NULL
otherwise. This means that we can create a function that will always work!
combining this with compact
which removes all NULL
values thus returning only the successful calls.
Reduce
purrr
includes an little group of functions called reduce()
(with its cousins reduce_right()
, reduce2()
and reduce2_right()
) which iteratively combines from the left (right for reduce_right()
) making
equivalent.
This example4 comes from Colin Fay shows how to use reduce()
.
This example by Jason Becker5 shows how to easier label data using reduce_right
.
pluck
I find that subsetting list can be a hassle more often then not. But pluck()
have really helped to alleviate those problems quite a bit.
head_while, tail_while
purrr
includes the twins head_while
and tail_while
which will gives you all the elements that satisfy the condition intill the first time it doesn’t.
rerun
if you need to do some simulation studies rerun
could prove very useful. It takes 2 arguments. .n
is the number of times to run, and ...
is the expression that have to be rerun.
compose
This little wonder of a function composes multiple functions to be applied in order from right to left.
This toy examples show how it works:
A more informative is found here6:
imap
imap()
is a handy little wrapper that acts as the indexed map()
. Thus making it shorthand for map2(x, names(x), ...)
when x have named and map2(x, seq_along(x), ...)
when it doesn’t have names.
or it could be used in conjunction with rerun()
to easily add id to each sample.
Sources
Overview
Purr Cheat Sheet
The goal of furrr is to combine purrr’s family of mapping functions withfuture’s parallel processing capabilities. The result is near drop inreplacements for purrr functions such as map()
and map2_dbl()
, whichcan be replaced with their furrr equivalents of future_map()
andfuture_map2_dbl()
to map in parallel.
The code draws heavily from the implementations of purrr andfuture.apply and this package would not be possible without either ofthem.

What has been implemented?
Every variant of the following functions has been implemented:
map()
map2()
pmap()
walk()
imap()
modify()
This includes atomic variants like map_dbl()
throughfuture_map_dbl()
and predicate variants like map_at()
throughfuture_map_at()
.
Installation
You can install the released version of furrr fromCRAN with:
And the development version from GitHub with:
Learning
The easiest way to learn about furrr is to browse thewebsite. In particular, thefunctionreferencepage can be useful to get a general overview of the functions in thepackage, and the following vignettes are deep dives into various partsof furrr:
Example
furrr has been designed to function as identically to purrr as possible,so that you can immediately have familiarity with it.
The default backend for future (and through it, furrr) is a sequentialone. This means that the above code will run out of the box, but it willnot be in parallel. The design of future makes it incredibly easy tochange this so that your code will run in parallel.
If you are still skeptical, here is some proof that we are running inparallel.
Data transfer
Dplyr Cheat Sheet
It’s important to remember that data has to be passed back and forthbetween the workers. This means that whatever performance gain you mighthave gotten from your parallelization can be crushed by moving largeamounts of data around. For example, if you are moving large data framesto the workers, running models in parallel, and returning large modelobjects back, the shuffling of data can take a large chunk of that time.Rather than returning the entire model object, you might consider onlyreturning a performance metric, or smaller specific pieces of that modelthat you are most interested in.
Purrr Map Cheat Sheet
This performance drop can especially be prominent if usingfuture_pmap()
to iterate over rows and return large objects at eachiteration.
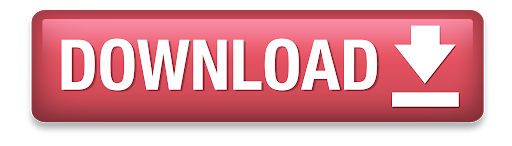